Development of Techniques for Solar Power Generation Prediction Using AI
- Tadashi Nakayama
- Ryohei Tomii
- Haruo Kita
- Yoshiki Sato
- Kohei Takada
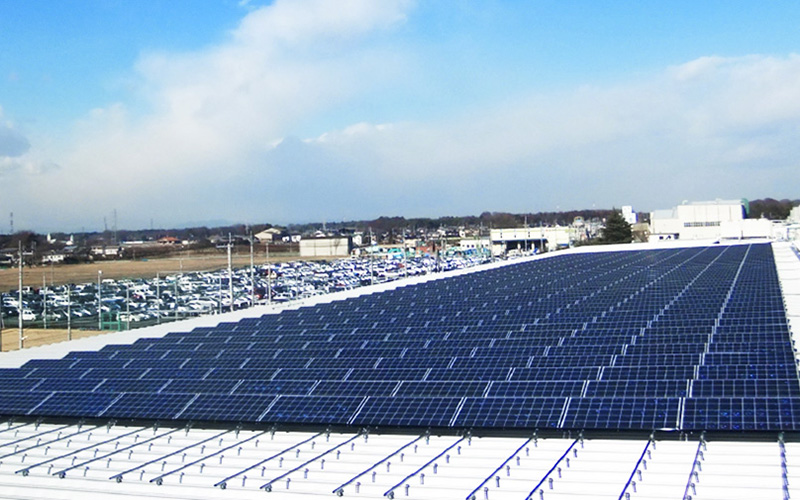
In order to promote carbon neutrality, the use of renewable energy that doesn't emit CO2 is expanding.
Among renewable energy resources, solar power generation is an important one which will continue to grow in popularity.
Solar power generation is a method of generating power depending greatly on the weather. In order for electric power companies to ensure the quality of electricity balancing supply and demand in the grid, businesses who operate solar power generation facilities may create a solar power generation plan and submit it to the electric power company in advance. This is because if the supply and demand of the power grid becomes out of balance, a possible result is a large-scale power outage. Additionally, each business must generate power according to the submitted power generation plan. If the power generation plan is drawn up with low prediction accuracy and the business fails to generate power according to the plan, possible consequences for the business are disadvantages such as penalties due to a difference between the plan and the actual amount of power generated and power being purchased at the market price. Therefore, highly accurate prediction of solar power generation is required for electric power companies and businesses to ensure the quality of electricity. Accordingly, a more accurate prediction technique is in demand.
To respond to these needs, Takaoka Toko has been studying various prediction techniques and systemization. It has recently developed two techniques that enable highly accurate prediction based on actual performance data on the amount of solar power generated.
Technology
Two Proprietary Techniques for Enabling Highly Accurate Prediction
The amount of solar power generated is strongly correlated with the amount of solar radiation. Given a known amount of solar radiation, it is possible to predict the amount of solar power to be generated; however, it is difficult to accurately predict the amount of solar radiation. Addressing this challenge, we have developed two original techniques that enable us to predict the amount of power to be generated with high accuracy even if the predicted amount of solar radiation contains errors.
One is a technique that uses Deep Learning (DL), which is a part of AI technology. We have developed a technique that aims to achieve higher accuracy of prediction with a larger volume of accumulated data on the actual amount of solar power generated. We have verified the technique, which made predictions with good accuracy on average. We expect that the accuracy will increase as we actually operate and accumulate actual performance data.
The other technique using a statistical approach enables prediction with short-term performance data. Verification has revealed that this technique has the advantage of being able to follow frequent changes in the amount of power generated.
With both techniques, we obtained results that can be more accurate than simply predicting the amount of power generated based on the amount of solar radiation.
Future tasks include identifying problems through demonstration experiments and proceeding with study of more accurate prediction techniques. Furthermore, we are also considering building and operating a system that incorporates demand forecasting.
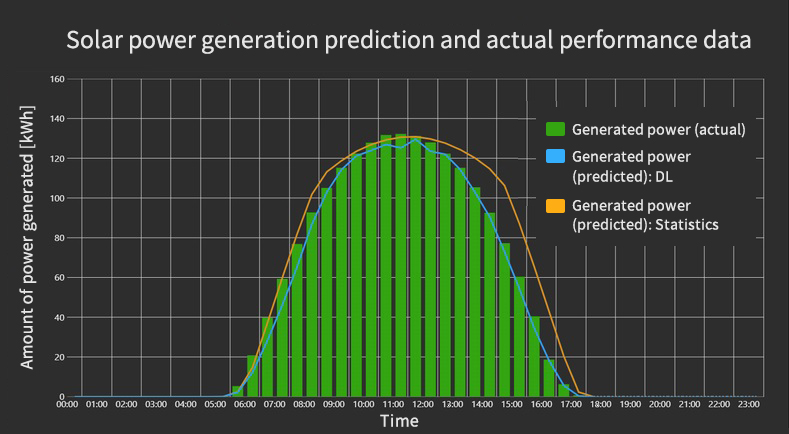
Profile
-
Tadashi NakayamaStrategic Technology Institute
Research & Development Center
ICT Technology Group
Deputy Section Manager -
Ryohei TomiiStrategic Technology Institute
Research & Development Center
ICT Technology Group
Deputy Section Manager -
Haruo KitaStrategic Technology Institute
Research & Development Center
ICT Technology Group
Chief -
Yoshiki SatoStrategic Technology Institute
Research & Development Center
ICT Technology Group -
kohei takadaStrategic Technology Institute
Research & Development Center
ICT Technology Group
Solar Power Generation Prediction Technology That Helps Ensure the Quality of the Power Grid
KitaBasically, at present, the prediction of the amount of solar power generated is based on a predicted amount of solar radiation. In the current project, the solar radiation prediction data is supplied by an external service provider. It is not pinpoint prediction data for the location of the power plant. In addition, the amount of power generated varies depending on how and in what environment the solar panels are installed. By overcoming these drawbacks, it should be possible to predict the amount of power generated with a higher degree of accuracy. We began to study prediction techniques based on this assumption. To sell generated power to a power company, solar power generation businesses are required to submit a power generation plan in advance stating the amount of power to be generated. Penalties will be imposed if the actual amount of power generated exceeds or falls short of the power generation plan; therefore, more accurate prediction is more beneficial for our customers (e.g. businesses operating solar power generation facilities).
NakayamaImproving the accuracy of power generation plans is not only beneficial to our customers, but it is also beneficial to the power grid by balancing supply and demand and ensuring the quality of electricity. If the power generation plan goes wrong and the power company's supply and demand balance collapses, in the worst case, a large-scale power outage may occur.
KitaWe studied various techniques during the project. In the end we presented two techniques, which were prediction using Deep Learning (DL) and prediction using a statistical approach. The technique using DL improves accuracy by accumulating past performance data. The statistical approach can make predictions even if actual performance data has been collected from a short period and the volume of accumulated data is small.
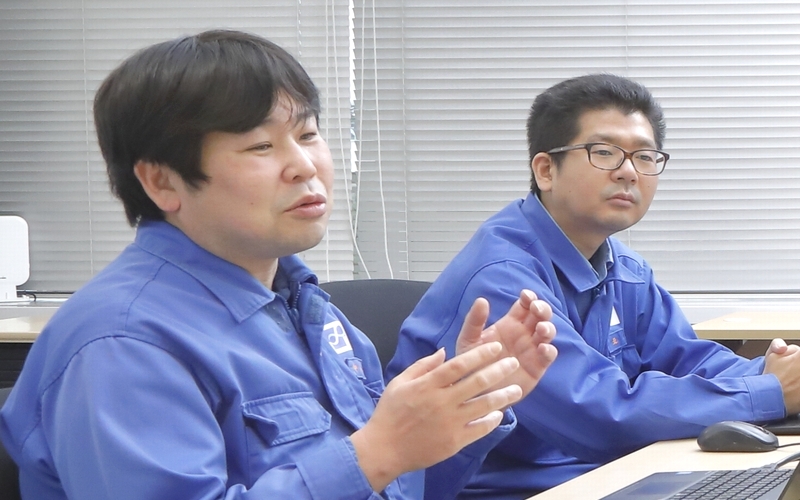
Starting from Scratch Followed by Repeated Trial and Error
KitaIn this research on methods using DL, predictions were made using past performance data. Real-world performance data included data obtained under special circumstances, so-called outliers. Applying them all may not give the desired result. It was very difficult to consider how to filter outliers and how to predict situations in which outliers appear.
SatoIn the study of the statistical approach, it was really difficult to devise a method for evaluating prediction accuracy (accuracy index) from scratch. Although several existing representative prediction accuracy indexes were available, applying them as they were caused various problems, such as failure to make predictions with stable accuracy when the amount of power generation was close to zero and inability to compare the accuracy between sites differing in the scale of power generation. Our first step was to find what kind of accuracy index would produce a plausible value. After many meetings and discussions among the members, we were finally able to create a universally usable index that was agreed upon by the parties concerned.
TakadaI built a system for the prediction algorithm developed by Kita and Sato to run on a cloud computing service. To be honest, I had no experience building a system in the cloud. Joining this project made me take the first step and proceed while learning. In this respect, it was a tough experience. Tomii had experience building a cloud system, so it was reassuring to receive a lot of advice from him.
TomiiWhen it comes to systematizing predictive algorithms, I advised you not to stop with a one-off project. We are also looking to develop this technology as a service, and we will continue to develop it horizontally. For example, if we could come up with a service that collects some kind of data, uses AI to make predictions based on that data, and provides the results to customers, I thought that the idea could be diverted to other themes other than solar power generation.
TakadaIn the project, we have a regular meeting once a week to share the report of the week and the plan for the next week among the members. Furthermore, by utilizing the chat tool introduced during the COVID-19 pandemic, we are frequently consulting among the members. By holding both online and offline meetings, we were able to build the system quickly. We could unveil it at the Takaoka Toko Solutions Fair (hereinafter referred to as the “fair”) held at the end of last year as planned.
KitaTomii is stationed in Kobe. Including him, we often work at different locations or go on business trips. Even in such circumstances, we use the chat tool to make quick responses. I think that the tool is contributing to the smooth progress of the project, and I am sure that it enables us to actively exchange opinions.
NakayamaThis solar power generation prediction project has been underway for about two years. Every step was new to the company. We started the project from scratch. As Takada said that it was his first time to build a system in the cloud, we really started it from scratch. During the development phase, it was a series of trial and error, and everyone had a really hard time. As a result of such efforts, we were able to build two highly accurate techniques in one system. Since the two techniques were published in Takaoka Toko Technical Report No. 9, we have already received inquiries and have ongoing meetings with customers. Also, in the fair, many people were interested in the system. The members who were at the booth on the day were bombarded with questions (laughs).
KitaI heard from a customer at the fair that solar power generation prediction in reality was based on empirical rules, referring to the same day a year ago. In contrast, the two techniques we studied in the current project both use actual performance data. We expect the two techniques to make predictions with a low error rate based on actual performance data and to produce more accurate and reliable predictions through repeated sessions. We have a plan to install the system at our customers’ sites as a demonstration experiment and intend to verify its accuracy by actually operating it.
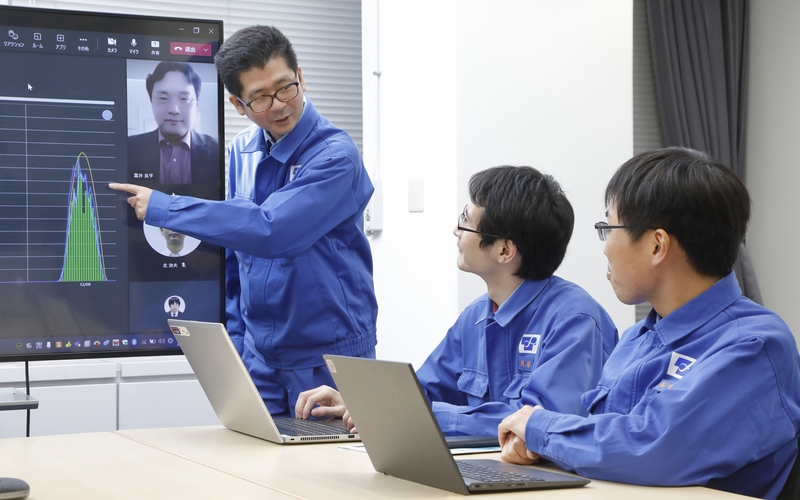
With an Eye on Changing Times and the Future
KitaCustomers who visited the fair gave us their views, suggesting our future challenges and goals. I would like to address them in the future. As Tomii said, application of the ideas cultivated in this project is not limited to solar power generation. I’m sure that the experience we have had in this project will be helpful when building systems that require some predictions in the future.
NakayamaThe world is moving toward the further spread of renewable energy. I think the challenge then is how to make efficient use of electricity, the amount of which depends on the weather. The point is whether or not to use conventional thermally generated power in conjunction with renewable energy or to purchase electricity from other electric power companies and how to use such electricity. One important factor in these considerations is prediction. So we will of course provide useful prediction technology to our customers. In the future, we will use our prediction technology to propose a system for helping customers use electricity efficiently.
KitaAs we aim to become a comprehensive energy service provider, we will definitely continue to address the use of renewable energy. We would like to utilize the technology that we have acquired through this project.
SatoIn addition to accumulating technical expertise, it was good for us to gain experience in following the cycle of study and verification. I believe that we have gained the ability to follow the cycle more efficiently and faster owing to repeated trial and error. I would like to make use of the experience not only in AI utilization but also in agile projects and many other undertakings.
TakadaIn recent years, use of a cloud computing service has been progressing in various industries. Takaoka Toko conducts its business mainly in the power industry, which is expected to use the cloud. We will probably build systems in the cloud on increasing occasions in the future. The experience we gained in the project will be of great help in this regard, and I personally want to further improve my technical skills in the future.
TomiiBy developing and providing systems in combination with the cloud, we can continue to develop and improve our product quality. A foundation for that way of thinking, in my opinion, has been created. I would like to spread this idea throughout the company and further improve not only the prediction quality of AI but also the quality and development level of the company’s manufacturing.
NakayamaAs a leader, I hope that the members who have gained experience in this project will play an active role in other projects as well in the future. In addition, this project is still ongoing. I will continue to work to make it a big one that will become a pillar of our business in the future.
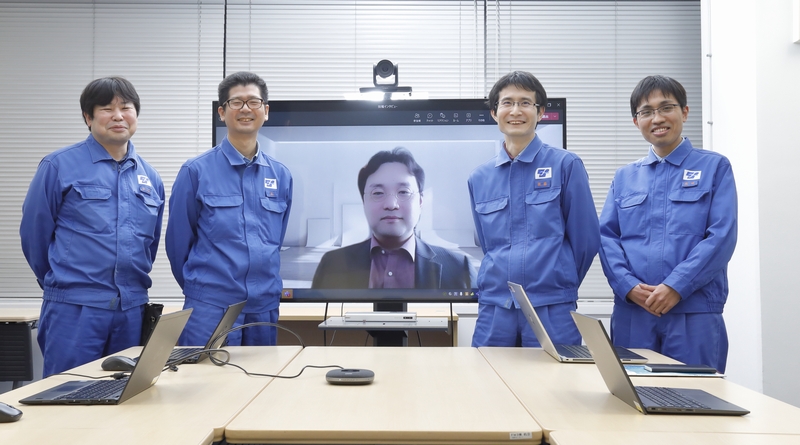
Latest articles
-
interviewProposal for new value via the first steel-tower-type full-color rendering illumination in JapanSince July in 2021, Prifoods Stadium in Hachinohe City, Aomori Prefecture has operated LED illumination equipment capable of the first steel-tower-type full-color light rendering in Japan. Takaoka Toko/Kyoya Denki/CADEC specific construction work consortium accepted an order for this equipment from Hachinohe City, and it was completed after nine months of work.
-
interviewBuilding a microgrid in Ueno Village, Gunma Prefecture, to Achieve Decarbonization and “Zero” Power Outages During a Disaster.The Sixth Strategic Energy Plan, a national policy, states that renewable energy is a main power source, and there is a need to expand the introduction of local power sources, including renewable energy. In recent years, the number of natural disasters in Japan has been on the increase, and a business continuity plan (BCP) is being emphasized for setting forth the methods and measures in advance to minimize the impact of an emergency and to ensure business continuity and a rapid recovery.
-
interviewRecycling technology of transformer oil aiming to achieve carbon neutralityNowadays, the work involved in carbon neutrality and the establishment of a recycling society is essential in business activities. The same trend is observed in the field of transformer oil. The international standard for the quality of transformer oil has been revised; there is now no distinction in quality between new transformer oil and recycled transformer oil (hereinafter “recycled oil”) abroad, which allows them to be treated equivalently.
-
interviewWeCharge: EV charging management system that can be operated in large parking areasEfforts intended to achieve carbon-neutral levels have been made increasingly more often, which requires expansion of the EV charging infrastructure in order to propagate electric vehicles (hereinafter called “EV”).